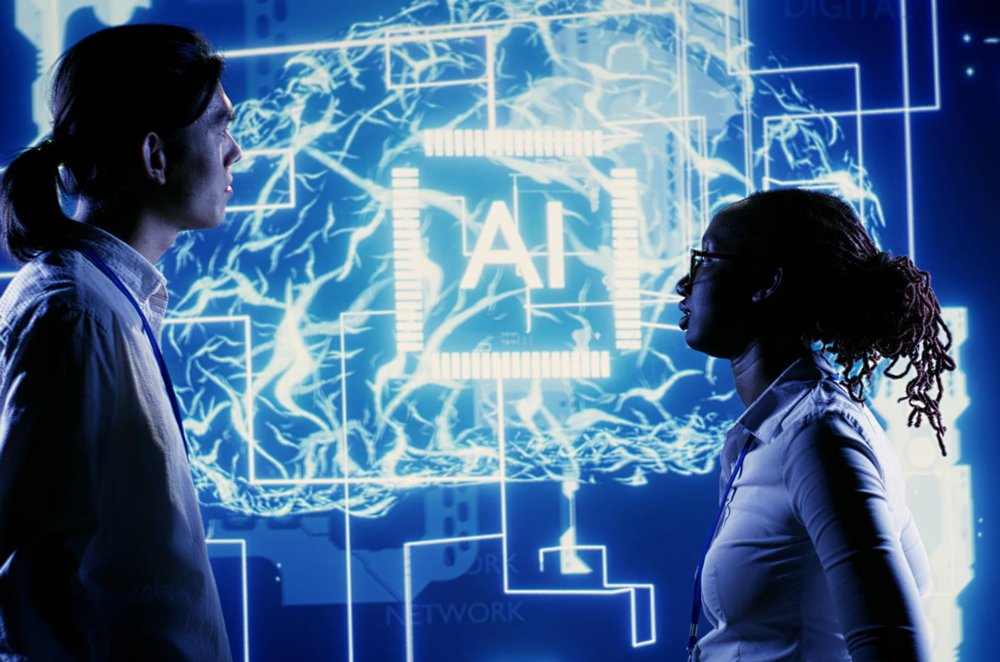
Cybersecurity Challenges in the Age of AI
Artificial Intelligence (AI) has become a cornerstone of modern technology, revolutionizing industries from healthcare to finance. However, as AI continues to evolve, it brings with it a new set of cybersecurity challenges. The integration of AI into cybersecurity strategies can bolster defenses, but it also introduces vulnerabilities that malicious actors are eager to exploit. Understanding these challenges is crucial for organizations and individuals striving to secure their digital environments.
The Double-Edged Sword of AI in Cybersecurity
AI offers significant advantages in the world of cybersecurity. It can analyze vast amounts of data at incredible speeds, identify patterns, and detect anomalies that might be missed by human analysts. However, the same capabilities that enhance security can also be leveraged by cybercriminals to launch more sophisticated attacks.
Key Cybersecurity Challenges Stemming from AI
1. AI-Powered Attacks
Cybercriminals are increasingly using AI to enhance their attacks. Machine learning algorithms can automate and optimize phishing schemes, making them more convincing and harder to detect. AI can also be used to identify and exploit vulnerabilities in systems at an unprecedented scale.
Example: AI-driven tools can generate highly personalized phishing emails by analyzing public data and social media profiles, making it easier to deceive even the most cautious individuals.
2. Adversarial Attacks on AI Systems
Adversarial attacks involve manipulating AI models to produce incorrect results. By feeding AI systems with specially crafted inputs, attackers can trick algorithms into making faulty predictions or classifications. This can compromise systems that rely on AI for security, such as intrusion detection systems or facial recognition technology.
Example: An attacker might subtly alter images to fool a facial recognition system, allowing unauthorized access to secure areas.
3. Data Poisoning
Data poisoning attacks involve corrupting the training data used to develop AI models. By injecting malicious data into the training set, attackers can degrade the performance of AI systems or cause them to behave unpredictably.
Example: An attacker could introduce misleading data into a model that detects fraudulent transactions, causing the system to miss actual fraud or flag legitimate transactions as suspicious.
4. Privacy Concerns
AI systems often require large amounts of data to function effectively. This raises privacy concerns, particularly when sensitive or personal information is involved. Mismanagement of this data can lead to breaches and unauthorized access.
Example: AI-driven analytics platforms might inadvertently expose personal data if not properly secured, leading to privacy violations and potential legal ramifications.
5. Ethical and Bias Issues
AI systems can perpetuate and even amplify existing biases present in the training data. This can lead to unfair or discriminatory outcomes in security applications, such as biased decision-making in identity verification or surveillance.
Example: An AI system used for hiring or loan approvals might unintentionally discriminate against certain demographic groups if it learns from biased historical data.
6. Increased Attack Surface
The integration of AI technologies can expand the attack surface of an organization. More AI-driven tools and systems mean more potential entry points for attackers. Managing and securing these systems requires comprehensive strategies and continuous monitoring.
Example: The deployment of AI in IoT devices creates numerous new endpoints that could be exploited if not adequately secured.
Strategies for Mitigating AI-Related Cybersecurity Risks
1. Robust Security Measures
Implementing strong security protocols, such as encryption, access controls, and regular security audits, is crucial for protecting AI systems and the data they handle.
2. Adversarial Training
Adversarial training involves exposing AI models to adversarial examples during the training process to improve their resilience against attacks. This can help mitigate the risk of adversarial inputs compromising system performance.
3. Data Integrity and Privacy
Ensuring the integrity of training data and protecting sensitive information are essential. Implementing data governance practices and privacy-preserving techniques, such as differential privacy, can help safeguard against data poisoning and privacy breaches.
4. Bias Mitigation
Regularly auditing AI models for bias and ensuring diversity in training data can help address ethical concerns and prevent discriminatory outcomes. Transparency in AI decision-making processes also supports accountability.
5. Continuous Monitoring
Regularly monitoring AI systems and updating security measures in response to new threats is vital for maintaining robust defenses. Employing AI-driven security tools can also aid in detecting and responding to emerging threats.
6. Collaboration and Information Sharing
Collaboration between organizations, researchers, and cybersecurity experts can help identify and address emerging threats related to AI. Sharing information about vulnerabilities and best practices contributes to a collective effort to enhance security.
Conclusion
AI is a powerful tool with the potential to both bolster and undermine cybersecurity efforts. While it offers significant advantages, it also introduces new challenges that must be addressed proactively. By understanding these challenges and implementing effective strategies, organizations and individuals can harness the benefits of AI while safeguarding against its risks. As AI technology continues to evolve, staying informed and adaptable will be key to maintaining a secure digital environment.